What is Machine Learning?
A statistical analysis where an algorithm learns from one data set to make predictions on a separate data set.
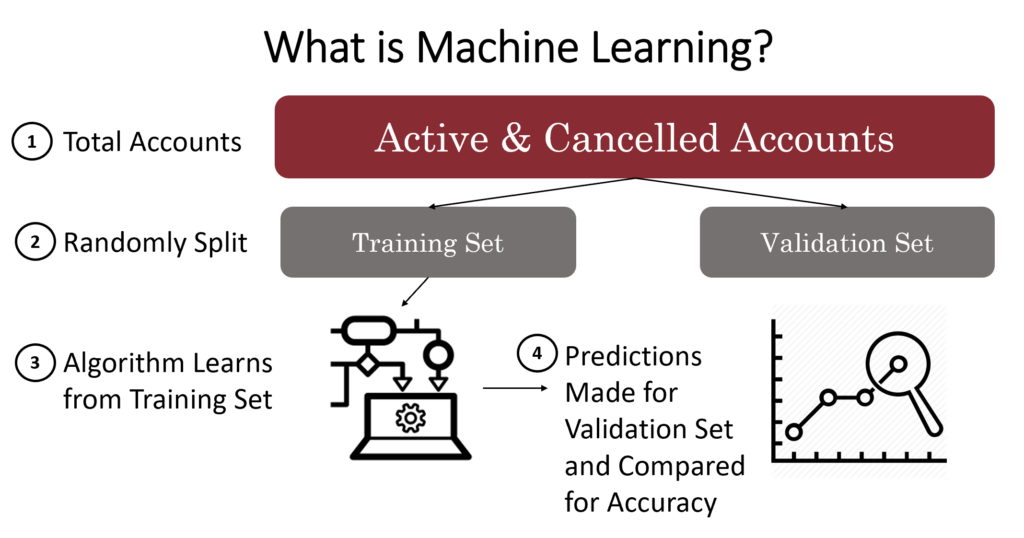
Machine Learning For Wine Club Retention
Objective: Identify Winery club members most at risk of leaving the wine club to administer preventative measures.
Methodology: BG Wine Consulting leveraged data from the Winery’s Point-of-Sale (POS) system and other Engagement Metrics to create a Machine Learning algorithm that predicts a club member’s likelihood of leaving the club in the next year.
The algorithm calculated a probability for likelihood of leaving and ranked all 538 members from least likely to most likely.
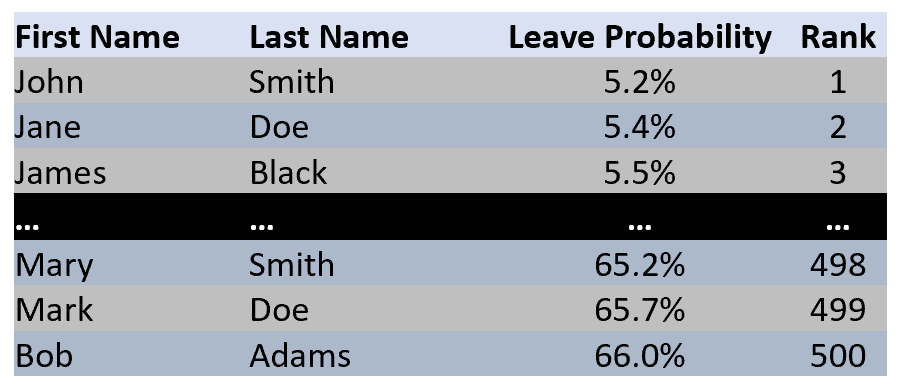
How Was This Data Used?
The Winery contacted the 50 people most likely to leave. They called each member to express their gratitude for continued membership and to see if there was anything the Winery could help them with such as customizing future shipments. The Winery also sent hand-written cards to those same members to further thank them for their membership.
Was The Machine Learning Prediction Correct?
Eight months after the analysis, BG Wine Consulting followed up with the Winery to see how accurate the analysis was.
We took the original predictions and grouped the 500 members into five tiers from Very Low Risk to Very High Risk of leaving. The Winery supplied the actual cancellations for each member since the initial analysis and indeed those members predicted to have the highest risk of leaving did leave in greater numbers.
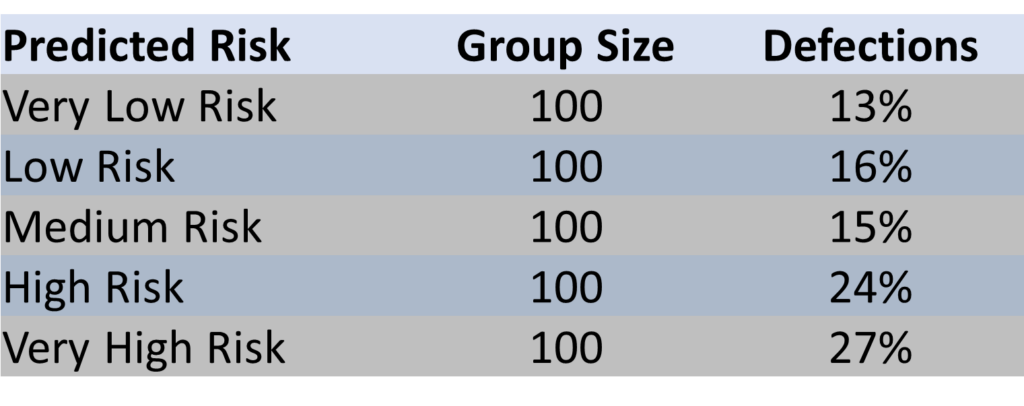
Did the Personalized Calls and Thank-You Help?
Actually, yes it did. Recall, the Winery contacted the 50 people most at risk. When we further break out the 100 people who were “Very High Risk” we see those that were contacted were directionally less likely to defect. While it didn’t eliminate the defections, it helped.

What is Next?
The Winery will continue to explore tactics to retain at-risk members. It is possible to re-run the machine learning model with the latest data to get up-to-date risk scores.
Additionally, BG Wine Consulting will continue refining the model to identify members in real time as their defection likelihood increases.